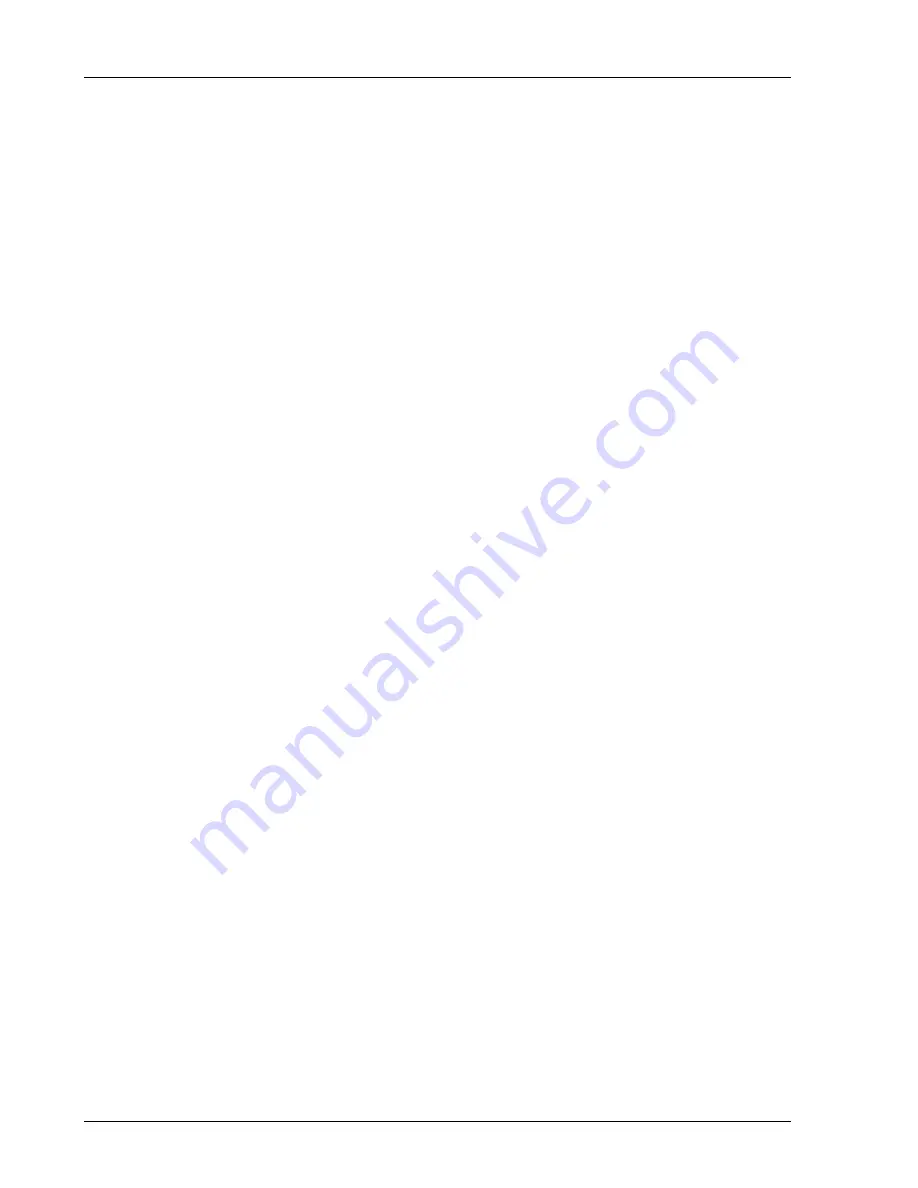
2-6 Analyzer
Basics
SR785 Dynamic Signal Analyzer
What is an FFT?
An FFT analyzer takes a time varying input signal, as you would see on an oscilloscope
trace, and computes its frequency spectrum. Fourier's basic theorem states that any
waveform in the time domain can be represented by the weighted sum of pure sine waves
of all frequencies. If the signal in the time domain is periodic, then its spectrum is
probably dominated by a single frequency component. The spectrum analyzer represents
the time domain signal by its component frequencies.
Why Look At A Signal's Spectrum?
For one thing, some measurements that are very hard in the time domain are very easy in
the frequency domain. Take harmonic distortion. It is hard to quantify the distortion by
looking at a good sine wave output from a function generator on an oscilloscope. When
the same signal is displayed on a spectrum analyzer, the harmonic frequencies and
amplitudes are displayed with amazing clarity. Another example is noise analysis.
Looking at an amplifier's output noise on an oscilloscope basically measures just the
total noise amplitude. On a spectrum analyzer, the noise as a function of frequency is
displayed. It may be that the amplifier has a problem only over certain frequency ranges.
In the time domain it would be very hard to tell.
Many of these types of measurements can be done using analog spectrum analyzers. In
simple terms, an analog filter is used to isolate frequencies of interest. The filtered signal
power is measured to determine the signal strength in certain frequency bands. By tuning
the filters and repeating the measurements, a reasonable spectrum can be obtained.
The FFT Analyzer
An FFT spectrum analyzer works in an entirely different way. The input signal is
digitized at a high sampling rate. Nyquist's theorem says that as long as the sampling rate
is greater than twice the highest frequency component of the signal, then the sampled
data will accurately represent the input signal (in the frequency domain). In the SR785,
sampling occurs at 262 kHz. To make sure that Nyquist's theorem is satisfied, the input
signal passes through an analog anti-aliasing filter that removes all frequency
components above 102.4 kHz. The resulting digital time record is then mathematically
transformed into a frequency spectrum using an algorithm known as the Fast Fourier
Transform or FFT. The resulting spectrum shows the frequency components of the input
signal.
The original digital time record comes from discrete samples taken at the sampling rate.
The corresponding FFT yields a spectrum with discrete frequency samples or bins. In
fact, the spectrum has half as many frequency bins as there are time points. (Remember
Nyquist's theorem.) Suppose that you take 1024 samples at 262 kHz. It takes 3.9 ms to
take this time record. The FFT of this record yields 512 frequency points, but over what
frequency range? The highest frequency will be determined by the period of 2 time
samples or 131 kHz. The lowest frequency is just the period of the entire record or
1/(3.9 ms) or 256 Hz. The output spectrum thus represents the frequency range from DC
to 131 kHz with 512 points spaced every 256 Hz.
Содержание SR785
Страница 4: ...ii ...
Страница 10: ...viii ...
Страница 80: ...1 64 Exceedance Statistics ...
Страница 158: ...2 78 Curve Fitting and Synthesis SR785 Dynamic Signal Analyzer ...
Страница 536: ...5 136 Example Program SR785 Dynamic Signal Analyzer ...