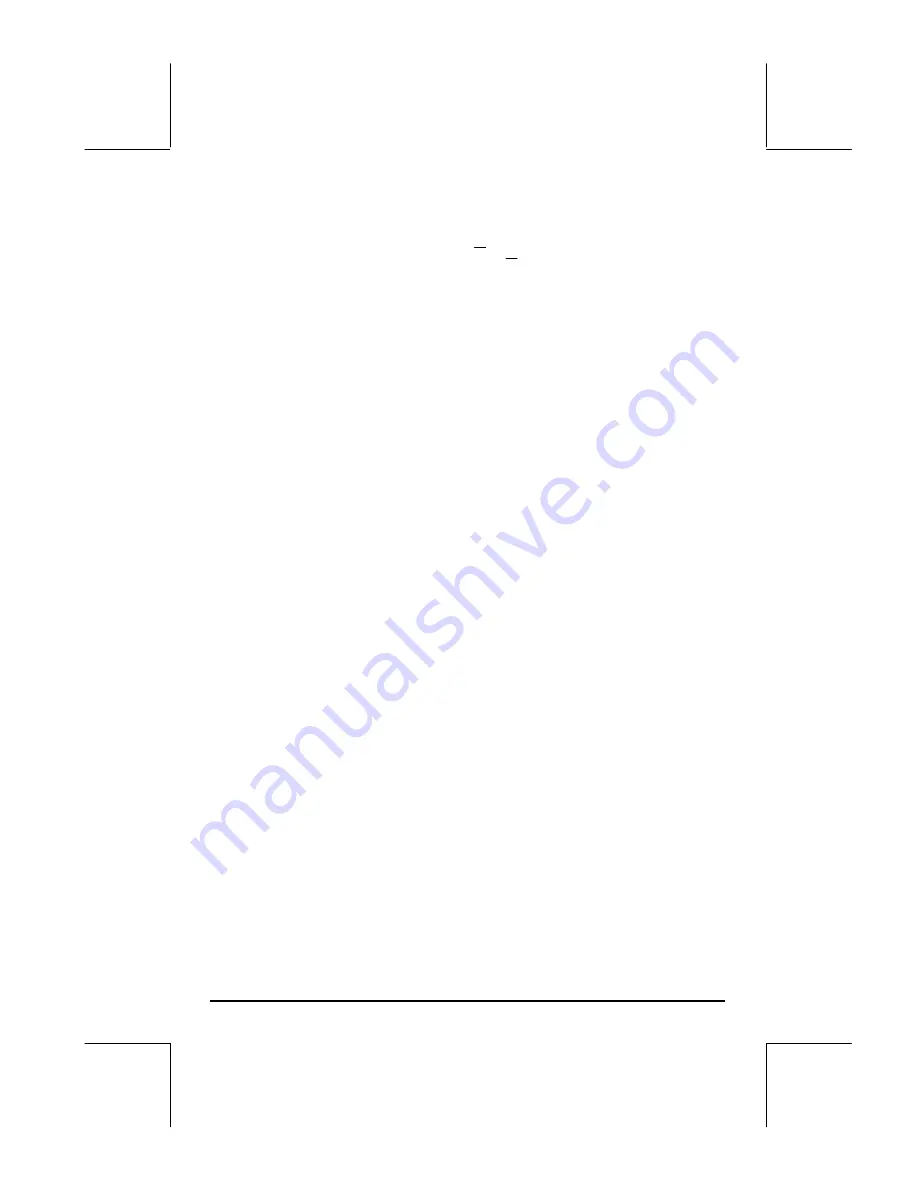
Page 18-23
to estimate is its mean value,
µ
. We will use as an estimator the mean value
of the sample,
X, defined by (a rule):
∑
=
⋅
=
n
i
i
X
n
X
1
.
1
For the sample under consideration, the estimate of
µ
is the sample statistic
x
= (2.2+2.5+2.1+2.3+2.2)/5 = 2.36. This single value of
X, namely
x =
2.36, constitutes a point estimation of the population parameter
µ
.
Estimation of Confidence Intervals
The next level of inference from point estimation is interval estimation, i.e.,
instead of obtaining a single value of an estimator we provide two statistics, a
and b, which define an interval containing the parameter
θ
with a certain
level of probability. The end points of the interval are known as confidence
limits, and the interval (a,b) is known as the confidence interval.
Definitions
Let (C
l
,C
u
) be a confidence interval containing an unknown parameter
θ
.
•
Confidence level or confidence coefficient is the quantity (1-
α
), where 0 <
α
< 1, such that P[C
l
<
θ
< C
u
] = 1 -
α,
where P[ ] represents a
probability (see Chapter 17). The previous expression defines the so-
called two-sided confidence limits.
•
A lower one-sided confidence interval is defined by Pr[C
l
<
θ
] = 1 -
α
.
•
An upper one-sided confidence interval is defined by Pr[
θ
< C
u
] = 1 -
α
.
•
The parameter
α
is known as the significance level. Typical values of
α
are 0.01, 0.05, 0.1, corresponding to confidence levels of 0.99, 0.95,
and 0.90, respectively.
Confidence intervals for the population mean when the
population variance is known
Let
X be the mean of a random sample of size n, drawn from an infinite
population with known standard deviation
σ
. The 100(1-
α
) % [i.e., 99%,
95%, 90%, etc.], central, two-sided confidence interval for the population
mean
µ
is (
X
−
z
α
/2
⋅σ
/
√
n ,
X+z
α
/2
⋅σ
/
√
n ), where z
α
/2
is a standard normal
variate that is exceeded with a probability of
α
/2. The standard error of
the sample mean,
X, is
⋅σ
/
√
n.